We are in the midst of a fundamental revolution in the ability to build models predicting outcomes involving humans. The developments in machine learning and deep neural network models estimation, coupled with rapidly increasing ability to input very, very large data volumes, has changed our lives.
These developments have the capability of greatly improving the efficiency of scores of transactions involving humans.
In the vast majority of cases the models depend on exposure to large amounts of data that inform estimation of the relative importance of different attributes. Early protocols for building such models harvested available data that the modelers could access. Facial recognition models scraped images of humans from available digital media. Predictive models for public health outcomes were based on browser search terms that were correlated with health outcomes. Police resource allocation models were built on data for arrest reports geographically.
All of these early efforts became now-favorite examples of failure. Google flu trends, predicting the course of the annual influenza spread, worked well for a while when searches for “achy joints,” “muscle pain,” “fever,” etc. held a stable correlation with flu outbreaks. When any societal event occurred that caused more healthy people to enter such searches, then the predictive power was sapped. When facial recognition software was based on digital media images from mainstream media, post-hoc it was learned that a very large portion of the images were of the then current president. The algorithm was really good at identifying one person but weaker on those not represented in the news. When police allocation was based on existing arrests by geography and time, it merely replicated the existing inequities in enforcement attention.
What do all of these failures have in common? They rely on existing data, most easily accessed by the modelers. Further, the modelers were sometimes quite naïve to the processes that produced the data. Finally, some models had little human intervention based on knowledge of the phenomena being predicted.
Contrast this to the regimens of most physical and social science data modeling. Statisticians have voiced the sentiment for years – “All models are wrong, but some are useful.” First, the scientific purpose usually involves some causal inference. Do the measured attributes, in some sense, cause the outcome attribute? Some of the models built to answer this question are as complex as those in the machine learning space. Scientists almost always are the strongest critics of the measurements that produce the data on which their models are based. They form this critical stance because they know the observations they can make are only proxies of what they want to measure. So they carefully design the observations/measurements to make the data as close to the target phenomena as possible. Then, when they model data that they themselves controlled, they scrutinize the models testing different assumptions about measurement errors in the data.
They have developed this regimen from lessons of failures of merely finding a convenient available source of data and building statistical models off the data. Predictive models that are not based on deep understanding of causal mechanisms will fail at some point.
Dealing with very high dimensional data with new computing tools will be an important part of our future. But the assumption that all existing data are sufficient to safely employ those tools for prediction is wrong. Only subject matter experts can inform what data we might be missing relevant to the causal mechanisms of some outcome.
Designing data for measurements not represented in already-existing data is the only way forward to create models that might be useful, even if they/re wrong. Assuming that all relevant data to improve global well-being will be organically produced as digital exhaust of human behavior is folly. Viva measurement!
Address
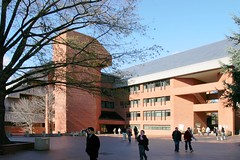
ICC 650
Box 571014
37th & O St, N.W.
Washington, D.C. 20057
Contact
Phone: (202) 687.6400
Email: provost@georgetown.edu
Office of the ProvostBox 571014 650 ICC37th and O Streets, N.W., Washington D.C. 20057Phone: (202) 687.6400Fax: (202) 687.5103provost@georgetown.edu
Connect with us via:
I think data design will leave its place to artificial intelligence in time. But I don’t know if we can see it.
Scary stuff
Another thought. In medical studies there is a phenomenon I think called The Heisenberg effect whereby by even doing the study itself you can affect the outcome ! Is there any similar issue with data input and analysis? Just wondering here.
As a followup do AI.programs in computers have an Heisenberg effect ? Crazy outa the box computer dumb question!
Interesting. Basically saying kinda garbage in garbage out ? We need to be smart about what we put in and why, and what we are looking for , and what’s the goal ! Sorta ? Good questions .