We are witnessing an interesting moment in use of artificial intelligence systems, especially those reflecting Large Language Models (LLMs) and generative features, like Chat GPT-4 and Bard.
Many of the issues of potential harm are well documented – bias due to poor training data, malicious use, privacy concerns, environmental impacts of data centers, and vulnerability to cyberattacks. Another concern that has less visibility is the violation of norms and values of users.
This latter is one of the issues that arise under the label of “safety” of LLM’s. Some of this work is attempting to reduce the output from LLMs that offend the user’s values.
What are the key issues regarding safety for AI in general? A new white paper from the Center for Security and Emerging Technologies addresses AI systems more generally and notes three key concepts, all focused on the nature of the learning algorithms. First, one challenge is building a system to self-assess the confidence with which it is making its prediction – sometimes called the robustness of the system. When the confidence is low, then it is desirable to have some fallback option or human intervention. Second, another desirable feature of an AI system is that humans are able to understand or interpret the behavior of the system. Ideally, this is an understanding of how new inputs to the system will inform its future predictions. Third, the term “specification” is sometimes used to measure the alignment of the AI performance to the goals of the designer.
This last seems relevant to generative AI applications with LLMs. Some developers note that initial post-training LLMs’ outputs are offensive to social norms and commonly-held beliefs and values of the likely users (e.g., racist, misogynistic, or aggressive output). It appears that common guidance is to have some review after the learning step is complete, either by a human or through additional algorithms. Additional training is then introduced to assure the given norms are followed in a revised platform.
It is this step that is interesting from a digital ethics perspective. Although is its referred to as a “safety” step, it is really the imposition of human values as perceived by those doing the evaluation. It occurs after the main content training is completed. However, what norms and/or values constitute the evaluation step do not appear to be widely-documented. It appears that for some cases, the developers and overseers of the original training step are conducting the evaluation. What values guided their decisions are largely unknown.
Even the developers often acknowledge that not all offensive behavior can be eliminated through these internal steps. Thus, after the release of the platform, users are commonly asked to report other examples of offensive or erroneous outputs.
What seems to be missing in the current environment is a community articulation of what values should be upheld in the performance of the platform and which are not to be policed. This might be viewed not as a post-construction patch, but a full design principle.
Further, most users are unaware of what additional evaluative steps have been executed to “clean up” the behavior of the platform. Thus, the change worth discussing is explicit statement and documentation of values, as well as their incorporation into the original platform design.
In one sense, the evaluative steps now in place are attempting to exercise community standards without community input. The values guiding the evaluation step are largely undocumented. They are patches after a design has been implemented. It seems like ripe territory for advances.
Address
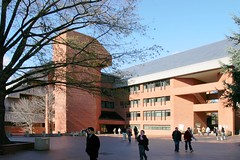
ICC 650
Box 571014
37th & O St, N.W.
Washington, D.C. 20057
Contact
Phone: (202) 687.6400
Email: provost@georgetown.edu
Office of the ProvostBox 571014 650 ICC37th and O Streets, N.W., Washington D.C. 20057Phone: (202) 687.6400Fax: (202) 687.5103provost@georgetown.edu
Connect with us via:
It might be helpful to require that AI output include explicit statements about the descriptive input/process, predictive input/process, normative input/process and prescriptive input/process upon which the AI output is based. In other words, a comprehensive statement concerning the AI algorithm should be included with the output (tantamount to a disclaimer or FYI).